How AI Can Solve Bottlenecks in the Lab and Beyond
Search For Schools
When you click on a sponsoring school or program advertised on our site, or fill out a form to request information from a sponsoring school, we may earn a commission. View our advertising disclosure for more details.
In the short amount of time since AI has been actualized, its powerful abilities in data analysis, pattern recognition, and prediction have been widely used to optimize business operations—revolutionizing fields like tech, finance, customer service, and manufacturing just to name a few.
Outside of corporate applications of the technology—which primarily serve to reduce business costs and fatten bottom lines—AI also stands to revolutionize the sciences.
In September 2023, academics from MIT, Harvard, and Stanford congregated at the National Advisory Environmental Health Sciences Council meeting to discuss how their respective fields like toxicology, environmental science, and epigenetics can harness the power of machine learning to solve the world’s most pressing humanitarian issues.
We talked to scientists about the current progress being made in their fields, what they envision for the future, and the potential obstacles ahead.
Meet the Experts
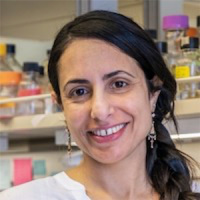
Shiri Levy, PhD is an epigenetic scientist passionate about how the study of protein design can uncover cures for diseases. She completed her master’s degree and PhD a thet Israel Institute of Technology before moving to Seattle to pursue a postdoctoral fellowship at UW, where she worked with the Institute for Protein Design to explore synthetic epigenetic modifiers.
She is currently an acting instructor at UW, where she lectures on stem cells in development and regeneration. In 2021, Dr. Levy founded Histone Therapeutics—a biotechnology company for developing drug modalities based on protein modifiers of chromatin remodeling, to create new options for treating disease.
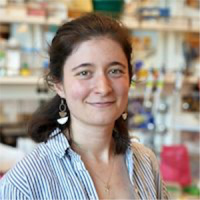
Clara McCurdy is a third-year graduate student at UW co-advised between this lab in the Institute for Stem Cell and Regenerative Medicine and the Institute for Protein Design.
Her focus is on using designed proteins to study signaling and direct stem cell differentiation.
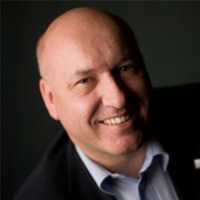
Thomas Hartung, MD, PhD is a professor at Johns Hopkins Bloomberg School of Public Health. He received his PhD from the University of Konstanz and his MD from the University of Tuebingen in Germany. The main objective of his work is to revolutionize toxicity testing with the underlying goal of improving public health.
His background lies in clinical and experimental pharmacology and toxicology, documented in more than 550 publications. Between 2002 and 2008, he served as Head of the European Centre for the Validation of Alternative Methods (ECVAM) at the EU Joint Research Centre.
Speeding Up Research in Epigenetics
Just over 50 years ago, during his Nobel Prize acceptance speech, American biochemist Christian Anfinsen described a future when one day, scientists would be able to predict the structure of any protein.
Scientists like Anfinsen were fixated on understanding this complex molecule—and for good reason. Proteins, often referred to as the building blocks of life, are not only essential for biological function but are central to the formation of many of the most confounding and deadly diseases.
Let’s take cancer, the leading cause of death worldwide and one of the most well-studied diseases. Tumorous cells can form when the instructions in our DNA are encoded incorrectly during cell formation, resulting in genetic changes or mutations. Proteins are central to this process; they are the worker bees that carry out the functions of cells.
Understanding the 3D shape of a protein matters because it determines a protein’s function. If scientists could accurately guess a protein’s shape and manipulate it, it would revolutionize our approach to medicine as we know it.
But in the absence of a crystal ball for predicting protein folding, the field of molecular design has lagged behind in its development. This is not due to lack of interest, but because of the sheer magnitude of the problem; the number of different ways a protein may fold into are nearly incalculable.
According to Levinthal’s paradox, a protein can theoretically morph into 10^300 different shapes. As Forbes reports, “it would take longer than the age of the universe for a protein to fold into every configuration available to it, even if it attempted millions of configurations per second.”
This didn’t stop computational biochemists from pressing forward, who started experimenting with different methods for determining a protein’s structure in the 1970s. Over the decades, progress was painfully slow and astronomically expensive—that is, until 2021 when there was a major breakthrough.
The Baker Lab at the University of Washington (UW) released a program RoseTTAFold Diffusion, that solved the structures of hundreds of proteins with a high level of success. Later the same month, Google’s DeepMind did the same for 350,000 proteins—nearly half of all known human proteins. Both were thanks to AI.
The advancement can’t be overstated. Now after decades, Anfinsen’s vision is being brought to fruition—with AI-based protein structure predictions winning the American Association for the Advancement of Science’s 2021 Breakthrough of the Year.
RoseTTAFold Diffusion (known as RFdiffusion for short) was made possible by machine learning, which is also baked into popular tools like DALL-E, the text-to-image generator that has quickly become popular on social media for its impressive ability to create original images based on a text prompt.
“Basically, the idea is that you can give [DALL-E] a prompt and it will output an image,” says Clara McCurdy, a third-year graduate student co-advised at the UW Institute for Protein Design and the Institute for Stem Cell and Return of Medicine.
“So you can give it ‘a corgi wearing a cowboy hat’ and it will come up with many different versions … because through machine learning, it’s learned what a corgi is, it’s learned what a cowboy hat is, and it’s been able to put those two together,” McCurdy explains.
In this way, DeepMind and RFDiffusion are similar to DALL-E. They produce plausible protein designs for a desired purpose much faster than the previous tools, requiring significantly less screening time.
Before these AI-based models, sometimes tens of thousands of designs would have to be tested in the lab before finding a single one that performed as intended.
“The [process] has gone from probably months to getting hits within a few weeks,” McCurdy says. “… Even just in that time [since] it has come out, it has totally changed the game.”
The ability to quickly design a protein with custom characteristics has allowed researchers like Shiri Levy, PhD, acting instructor in Stem Cells in Development and Regeneration at UW, and her colleagues to take the next logical step.
In 2022, Dr. Levy and her colleagues used an AI-designed mini-protein that would bind to PRC2—a protein complex known for its involvement in cancer. Previously, researchers could block PRC2 with chemicals, but the method lacks precision and would end up affecting PRC2 function throughout the genome.
“We learned that in cancer, PRC2 represses many of the genes that are supposed to stop the cell cycle from happening,” says Dr. Levy.
“So think of a car that has the gas pedal on all the time and the brake pedal is completely silenced and shut off. What we wanted to do is … take the repressive mark and lift it off the brake so we can upregulate the gene expression,” Dr. Levy explains.
The project was a success; the team showed they were able to block PRC2 and selectively turn on four different genes. “Let’s think about a chemo drug that a cancer patient takes,” says Dr. Levy. “[Chemo] spreads all over their body and goes across multiple pathways and multiple targets.”
But if new proteins were designed to bind and inhibit only cancer-causing proteins or target specific molecular pathways involved in cancer progression, it would mark the beginning of a new era in medicine.
The technique can theoretically be applied to many diseases caused by cellular mutations, such as a chronic type 1 diabetes patient with an insulin gene mutation: “You can give insulin to a [diabetes] patient, and they will survive for a long time, but … at the end of the day, their pancreas doesn’t make insulin, and that person needs to be injected every single day. We want to take it all the way, to make that home run, and fix the pancreas to make insulin on its own.”
While Dr. Levy and her colleagues worked specifically on the PRC2 complex, there are many other proteins that scientists can use AI to target.
For instance, NYU Grossman School of Medicine and the University of Toronto are producing customizable zinc finger proteins—one of the most common protein structures that regulate gene expression—with its own new AI-based program called ZFDesign.
In the future, researchers hope this approach will be useful for diseases with multiple genetic causes, such as heart disease, obesity, and autism. “The dream is to control the cell cycle … and by that, fix a disease forever,” says Dr. Levy.
Innovation in Toxicology and Pharmaceutical Testing
Thomas Hartung, MD, PhD professor at Johns Hopkins Bloomberg School of Public Health, has dedicated his career to forwarding a paradigm shift in his field.
He says that AI is helping to harness billions of toxicology-related data points and extrapolate them in a way that was never feasible before.
Animal testing serves as a great example of the progress being made with AI. The method has long been used in pharmaceutical research to test if substances will be toxic for humans. The process is archaically straightforward: create a new chemical or drug and use it on an animal to find out if it’s safe and effective.
While the ethical questions of animal testing are obvious and have long been debated, that’s not the only reason academics like Dr. Hartung would like to find alternatives. Increasingly, research suggests that animal models are unreliable predictors of drug safety in humans.
There are some harrowing cases in which people were harmed in the clinical testing of drugs that were previously found to be safe in animal studies—prompting researchers to look into other methods.
For example, in 2006, six men took a drug known as TGN1412 intended for the treatment of autoimmune diseases and leukemia during a clinical trial in London. Within a couple of hours, all six men had disastrous reactions including organ failure and brain swelling. However, the same drug had previously been given to monkeys at a 500-fold higher dose, which didn’t indicate any safety issues whatsoever.
This event, known as the Elephant Man drug trial, caused a shockwave in the scientific community, raising questions about the accuracy and reliability of animal testing in toxicology. Within the last decade, researchers have begun to explore the idea of creating tools that would use existing research to predict how a drug might affect humans.
Dr. Hartung and his colleagues took the task upon themselves, creating a database with about 10,000 chemicals and 800,000 associated studies to see if they could beat the accuracy of animal testing by leveraging already available data—and they succeeded.
The model predicted 190,000 cases where a chemical was classified by regulators as 87 percent correct, while the respective animal tests were only 81 percent reproducible.
The paper was downloaded several ten thousand times, covered in Science and Nature as well as more than 200 press outlets, says Dr. Hartung.
In another study published in 2022, researchers showed that the method can predict human skin sensitization 82 percent correctly compared to the best animal test, which achieves 74 percent accuracy.
“These examples illustrate the potential of these approaches,” says Dr. Hartung. “A number of follow-up studies were published, and currently a $20 million E.U. project (ONTOX) is further developing the approach.”
While there is still more headway to be made, the method is promising. In 2023, a new US law was passed that lifts the FDA’s hard requirement for pharmaceutical companies to test new drugs on animals before human trials. The landmark law amends an old rule by the FDA that was originally passed in 1938.
While it’s unlikely that animal testing will ever be completely abolished, the move represents the validity of different drug testing methods and could signal a future where animal testing is significantly minimized.
Tackling Climate Change
Outside of advancements in toxicology and medicine, scientists are also finding ways to use the power of machine learning to solve the climate crisis.
One way is through AI-powered sensors and imaging systems, which are being used to collect more data—and better data—on the environment.
A 2022 paper that looked at how image-capturing autonomous drones can be used to take inventory of forests in southeast Queensland found that the drones could catalog more accurate data, such as tree height, compared to old-school, laborious methods for field assessment.
Additionally, previous methods could take days or weeks, depending on the size of the surveyed area, whereas with drones, data delivery is almost instantaneous.
Scientists can expand use of this method to keep a closer eye on commercial deforestation activities. At present, determining if logging is legal or illegal is a major issue. One 2021 study estimated that nearly 94 percent of deforestation activities in the Amazon and the Cerrado savanna of Brazil are illegal. With AI-enabled drones, the government could more easily enforce rules.
Additionally, AI drones could be used to increase the sustainability of farming operations and in turn, reduce related emissions. For instance, faster and more accurate monitoring can help farmers react faster to crop-destroying insects and weather events—drastically minimizing the high level of waste associated with farming.
Due to the exciting potential, the AI in agriculture market is expected to grow from $1.37 billion in 2022 to $11.13 billion by 2032.
With these new technologies at work, environmental scientists can also leverage AI in the next step of their research: to improve analyses and garner more meaningful conclusions.
For example, in 2023, a research team from Pennsylvania State University sought to study air quality in different urban regions in the U.S. by letting AI recommend which mathematical models were the best-suited for analyzing each geographic region.
The researchers found that the technique improved the accuracy of measurement by an average of 17.5 percent. They hope their research can help public health officials zero in on air quality pollution hotspots and take more meaningful political action.
This application of AI could also be used to improve research on water quality, temperature, and greenhouse gas emissions and ultimately, to optimize waste management strategies to curb environmental impact.
Obstacles Ahead
Over the last year, conversations about concerns with AI such as data privacy, disinformation and racial bias have played out publicly with companies like OpenAI at the center. Legislators have struggled to answer questions about how to regulate AI and in which circumstances it should be controlled.
“The key problem is that [regulators] are … not literate in these new methods,” says Dr. Hartung. “The rapid advancement does aggravate the situation.”
At present, a point of contention is black box problem—which refers to the fact that the internal workings of AI, specifically deep learning models—are often a mystery to scientists and programmers.
If the researchers themselves can’t tell how or why an algorithm came to a certain conclusion, how can regulators trust it? It’s a worthy question, Dr. Hartung acknowledges. If black box issues go unaddressed, regulators could pump the brakes.
“I think everyone sees the potential and does not want to miss [it], but regulation—for good reason—is conservative. Safety of patients and consumers is at stake,” he says.
The problem, however, is not insurmountable. The key will be to build data transparency into the architecture of future algorithms, of which Dr. Hartung is a staunch advocate.
But if this is the biggest speed bump on AI’s road to success, the outlook is promising. With some of the biggest bottlenecks in progress resolved, pressing issues in environmental science, epigenetics and medicine could be next.